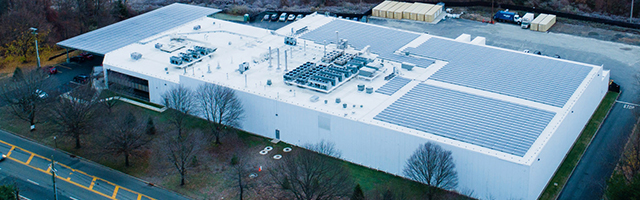
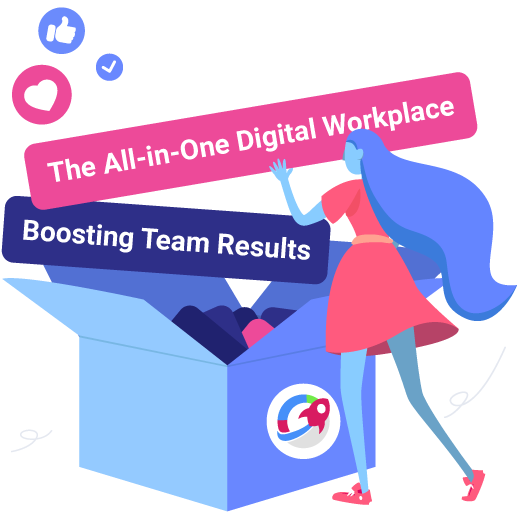
What Is Predictive Analytics And Why It Matters?
Predictive analytics is a type of data analysis that is used to predict events. In this blog, we explain how does it work, what are the main predictive analytics techniques, what are its benefits for businesses, and much more + examples.
In the business world, analytics help organizations optimize their internal and external processes, expand, and ultimately increase their profitability. Some types of data analytics focus on helping users understand what and why things have happened, while other types of data analytics focus on providing insights into what could happen in the future. This is the concept of predictive analytics, a data analytics tool that businesses use to identify risks and opportunities and guide their decision-making.
What Is Predictive Analytics?
Predictive analytics is a type of data analysis that is used to predict events. It looks at current and historical data patterns to determine whether any of those patterns are likely to emerge again and then uses statistical algorithms and machine learning techniques to identify the likelihood of future outcomes. This allows businesses of any size and industry to adjust where they use their resources, improve operational efficiency, reduce risk, make strategies based on facts, and guide their decision-making process.
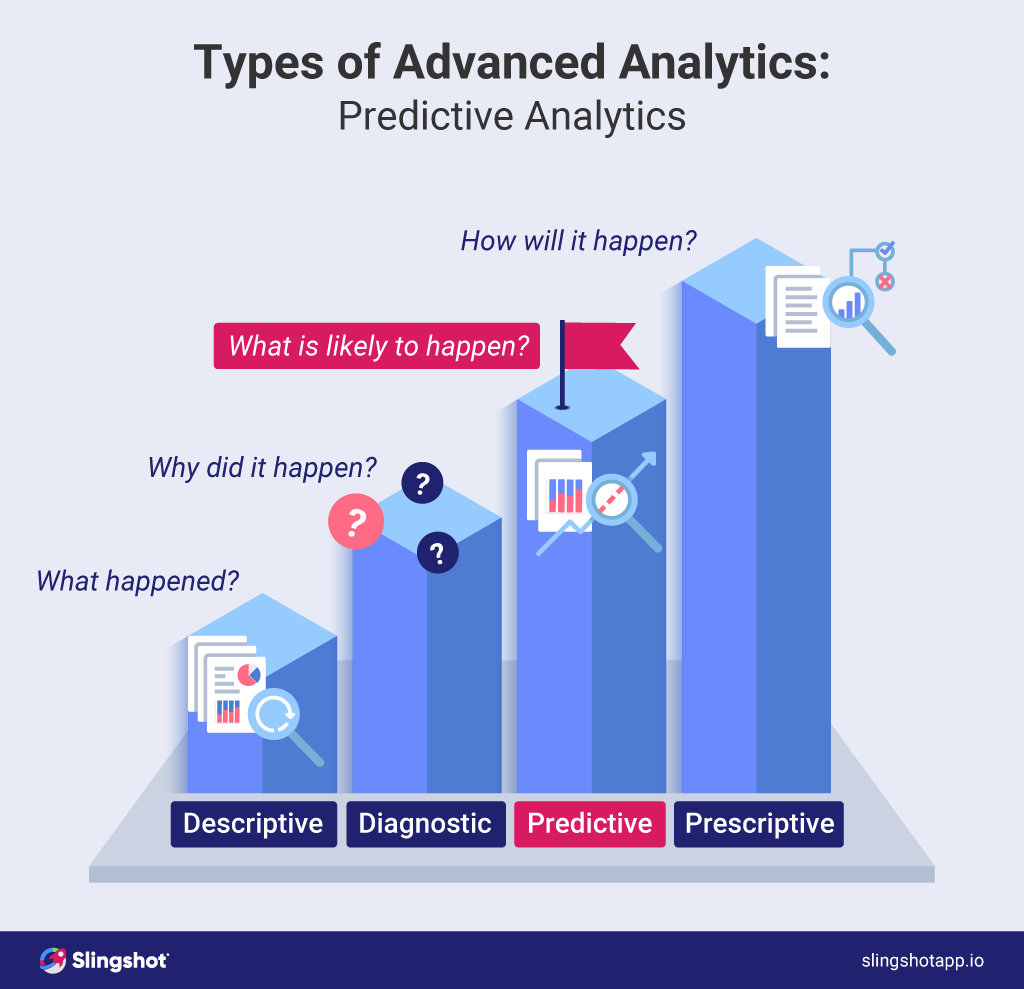
How Does Predictive Analytics Work?
When working with predictive analytics, the first step is to identify the problem that you’re trying to solve and what your project will cover. From here on, there is a systematic flow that the predictive analytics process follows:
- Project definition – The most crucial aspect of the project definition is understanding the goal of your predictive analytics project. What questions would you like to answer? Do you know the type of events or outcomes that you want to predict? Knowing the answers to these questions will help you get the right value driver from this initiative.
- Data collection – Predictive analytics is most effective when you can leverage a large volume of data for slicing and dicing, and to run necessary algorithms. If your organization has defined processes to collect data, then there shouldn’t be any challenge in accumulating it. But if it doesn’t, you may have to configure a data mining or data aggregation tool to harvest the data from your organization. Determining how to source data should be part of your project definition.
- Data cleaning – Before you start analyzing your data, it is important to clean the data for effective analysis. The cleaning process involves the consolidation of data from multiple sources into a single database and ensuring that data is formatted consistently.
- Deep data analysis – The goal of the data analysis is to reveal trends and patterns in the data and to use that information to create predictive models that can depict future actions. This step involves conducting two general methods for data analysis – statistical regression methods and machine learning techniques.
- Model construction – Once your data has been processed and analyzed, you can start building a predictive model for anticipating future events. Multiple models will be generated, and you have to select which one is the best (most accurate) for predicting your project’s future events.
- Deployment – The next step is to deploy the selected model into everyday use. Everyday use is again linked to the project definition and your initial goal. For example, if the model is used in the telecommunication industry to predict network usage, then this model must be put into use for monitoring real-time service performance and coming up with reports of possible slow performance or connectivity issues.
- Monitoring – Once the model is deployed, it needs to be constantly monitored and reviewed to ensure effectiveness. New data can be integrated as it becomes available to support the model on an ongoing basis.
Predictive Analytics Techniques
Predictive analytics relies heavily on complex models/techniques that have been designed to make inferences about the data it encounters. These predictive analytics techniques utilize algorithms and machine learning to be able to analyze historical and current data in order to predict future trends.
There are three main techniques used in predictive analytics: decision trees, regression, and neural networks.
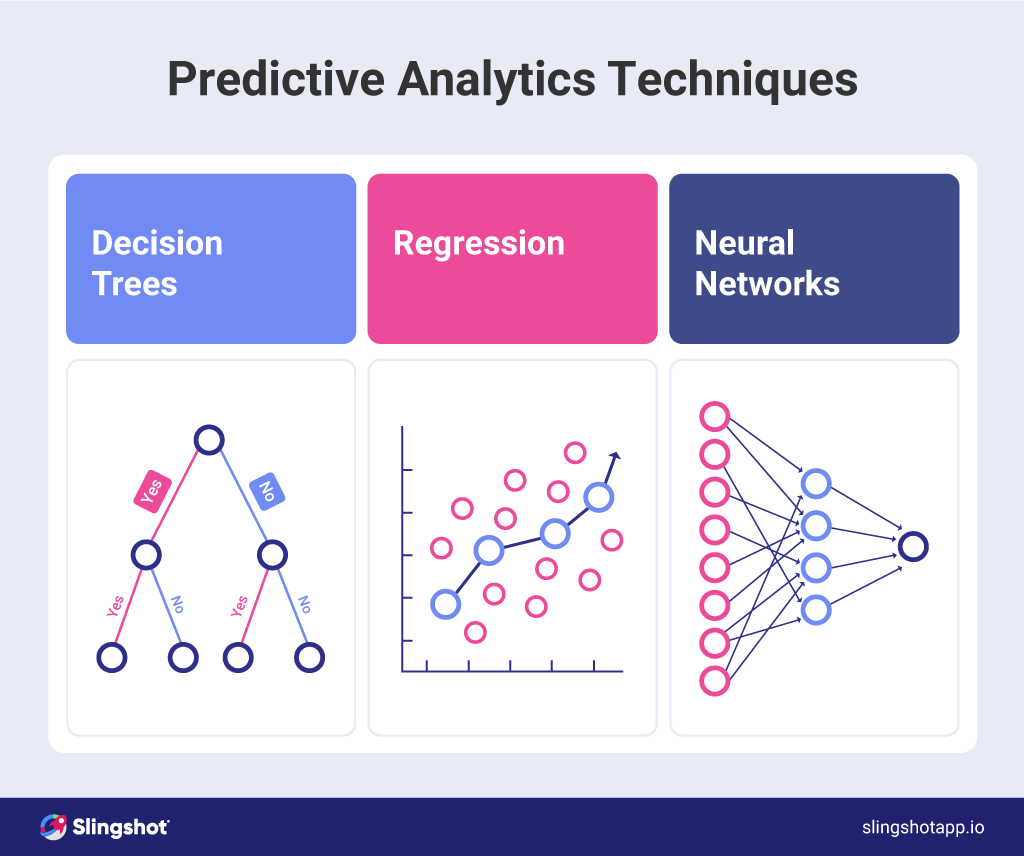
Decision trees – this is one of the best predictive analytics techniques because it handles missing values and is simple to comprehend. The decision trees use branching to visually show possibilities stemming from each outcome or choice. Each branch is a possible decision between two or more options, whereas each leaf is a classification – a yes or no.
Regression – there are three regression techniques for different scenarios. Different data questions require different applications of regression, but generally, the predictive analytics regression technique assists with understanding relationships between variables. Linear regression is used if only one independent variable can be ascribed to an outcome. Multiple regression is used if multiple independent variables have an effect on an outcome. And logistic regression is used when the dependent variable is binary.
Neural networks – are the most complicated predictive analytics technique. It utilizes algorithms to figure out possible relationships within data sets. Neural networks employ AI, thus allowing more sophisticated pattern recognition.
Why Is Predictive Analytics Important?
Nowadays, data is one of businesses’ most valuable assets. Data analytics is extremely important in the business world, transforming it in multiple ways. Analytics is helping businesses to survive in the market.
Predictive analytics, specifically, is important because it lets organizations accurately predict what is likely to happen and make critical decisions based on that data. Lots of businesses today fail to survive because of their inability to forecast and therefore plan and execute successful business strategies.
Businesses that make their key decisions based on intuition or guesswork have lost significant time and money failing to launch products that their target customers want or need. On the other side, businesses using predictive analytics to drive their decision-making gain a competitive advantage. Predictive analytics enables organizations to outperform their competition by capitalizing on new opportunities.
Predictive Analytics Benefits
There are numerous benefits to using predictive analytics. These are just a few of the best:
Improving Decision-Making
By analyzing historical data and industry trends, predictive analytics helps organizations make informed predictions about what the future could hold for them and therefore formulate strategies based on likely scenarios. This predictive capability allows all business users to make smarter decisions backed by facts. Additionally, data-driven decision-making improves productivity and increases the confidence of your team.
Detect Fraud
Predictive analytics can identify patterns to detect and prevent fraudulent behavior. As cybersecurity becomes a growing concern, predictive analytics examines all actions on a network in real-time to spot abnormalities that may indicate cyber vulnerabilities and threats. This helps businesses put relevant procedures in place and keep their data safe.
Reduce Risk
With the help of predictive analytics, analysts can forecast potential areas of risk and identify how these risks may affect their business. This finds use in many different industries, but perhaps it’s most beneficial for finance and insurance companies. For example, credit scores are a well-known example of predictive analytics. Thanks to this, financial professionals can determine the creditworthiness of an individual and make more confident decisions regarding whether the borrower will honor his debt obligation.
Reduce Costs
Predictive analytics can also help you reduce operational costs by helping you make more informed decisions about when to make new orders, when to invest more in your marketing campaigns, or how to manage your inventory and price your products.
Improving Efficiency in Operations
One of data analytics’ most significant strengths is in improving the efficiency of operations. Many organizations use predictive analytics to streamline workflows, manage resources and optimize performance. Data helps them reveal where they can improve, how they can do that, and optimize their overall processes and performance for maximum profitability.
Identify New Product/Service Opportunities
As predictive analytics in its essence predicts the future, it is of great importance when it comes to developing new products/services.
‘’Predictive analytics in product development has the potential to sharpen forecasts about product performance, product failure, and downtime, thus generating incredible value to organizations,’’ says Amit Joshi.
Predictive VS Prescriptive Analytics
Prescriptive analytics is the most advanced form of data analytics. It combines the insights from the descriptive analytics process, which provides insights on what happened, the diagnostic analytics process, which provides insights on why it happened, and the predictive analytics process, which provides insights on what could happen. This way analytics users can anticipate what will happen, when it will happen, why it will happen, and how.
Both predictive and prescriptive analytics are components of business data analytics, and both are used to inform and guide your business strategies and decision-making process based on collected data. The main difference between the two is that predictive analytics forecasts potential future outcomes, whereas prescriptive analytics helps you draw up specific recommendations for the optimal next step.
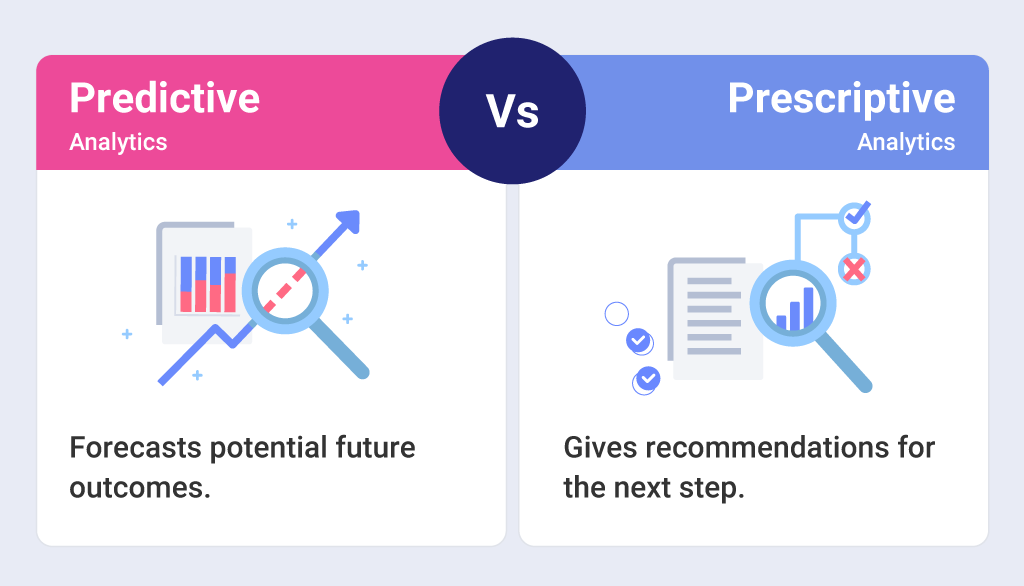
But you shouldn’t rely solely on just one or the other. When used in conjunction, both types of analytics can help you create the strongest, and most effective business strategy possible. It is great to know what could happen in the future, but if you don’t identify what your next step should be to make the most out of this knowledge, then you’ll be missing plenty of opportunities and won’t be able to achieve optimal results.
Predictive Analytics VS Machine Learning
A common misconception is that predictive analytics and machine learning are the same things. Well, they aren’t although we understand why some people may think so. Let us explain.
By reading until here, you already know what predictive analytics is – simply put, it is an analysis process that helps us identify and understand possible future outcomes. At its core, predictive analytics uses a series of statistical techniques, including machine learning. On the other hand, machine learning is a subset of Artificial Intelligence (AI) that enables computers/systems to learn without explicit programming. Machine learning uses algorithms to predict new output values through the usage of historical data.
So, what exactly is the difference between predictive analytics and machine learning? Both play a crucial role in helping organizations cultivate a data-driven culture and shape up better and smarter business strategies. However, where machine learning is heavily coding-oriented and its primary role is to let computers learn automatically without human assistance and adjust their actions according to their learnings, predictive analytics still rely on human intervention. Predictive analytics needs data analysts to determine and test the correlations between cause and outcome.
Predictive Analytics Examples
Any industry can use predictive analytics to optimize operations, reduce risks, develop better and more effective marketing and business strategies, reduce costs, increase revenue, and of course, forecast outcomes. Here are a few examples of how predictive analytics is used in different industries:
Healthcare – healthcare organizations, hospitals, and doctors use predictive analytics in several different ways, including intelligently simplifying internal operations, polishing the utilization of their resources, and improving care teams’ coordination and efficiency. But most importantly, predictive analytics allows them to identify patients at risk and the development of diseases.
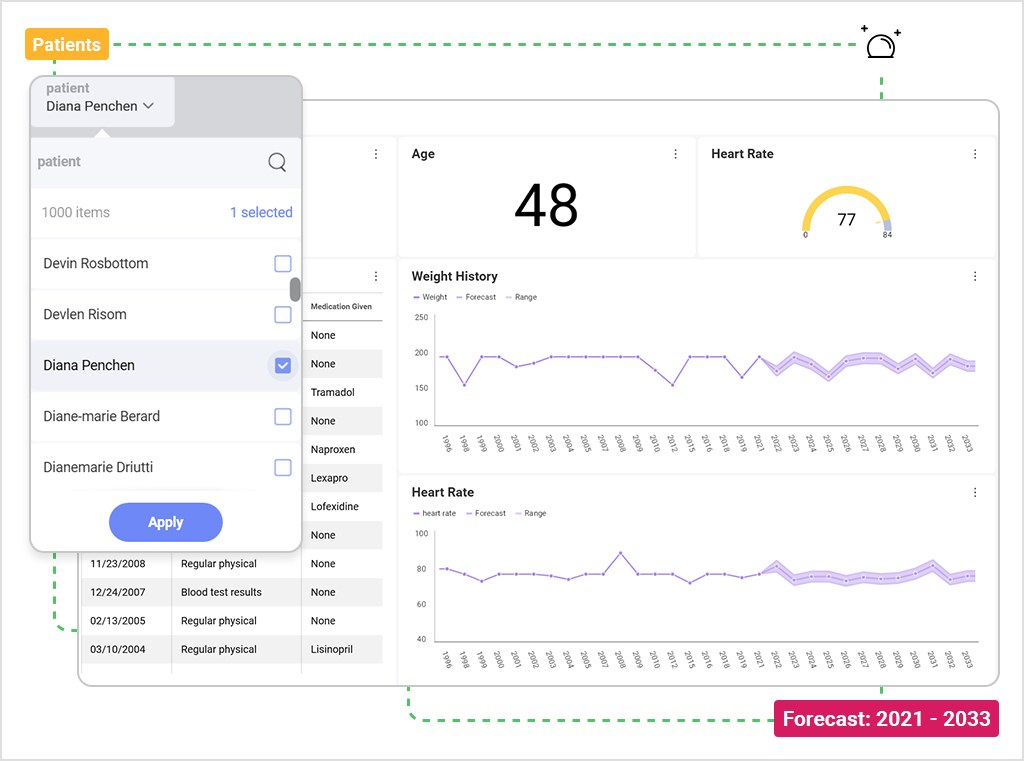
Manufacturing – for manufacturers it’s very important to reveal the factors leading to production failures and reduced quality, as well as optimize parts, resources, and distribution, thus they use predictive analytics to monitor supplier performance, predict maintenance requirements, optimize production capacity, and more.
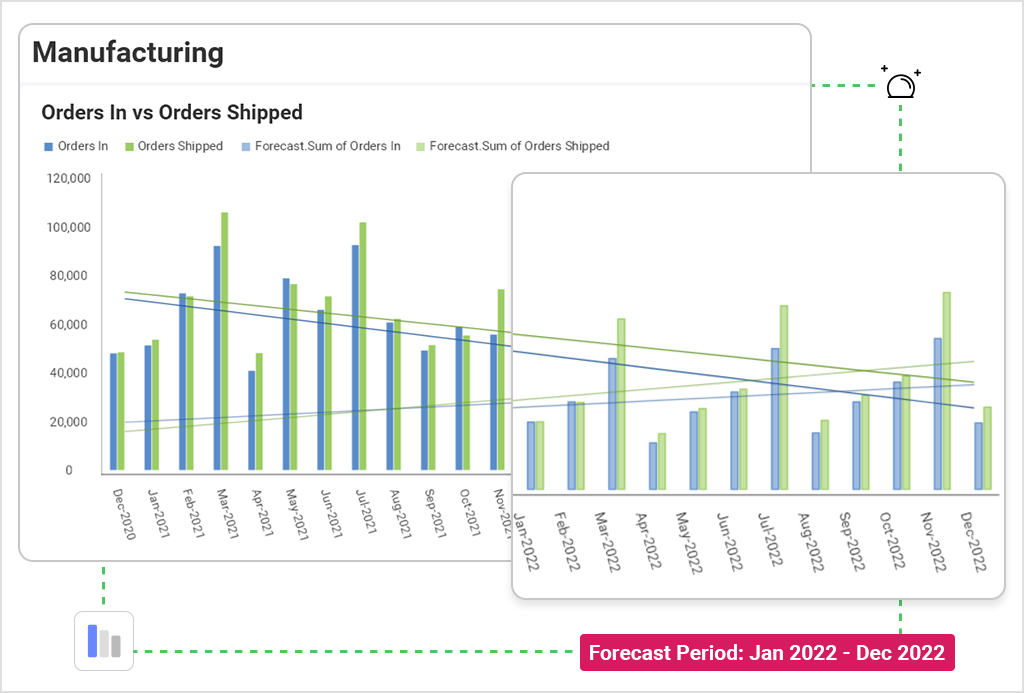
Retail – retailers use predictive analytics to forecast future sales and demands and optimize their pricing strategies, analyze the effectiveness of promotional events, and determine which offers are most appropriate for consumers.
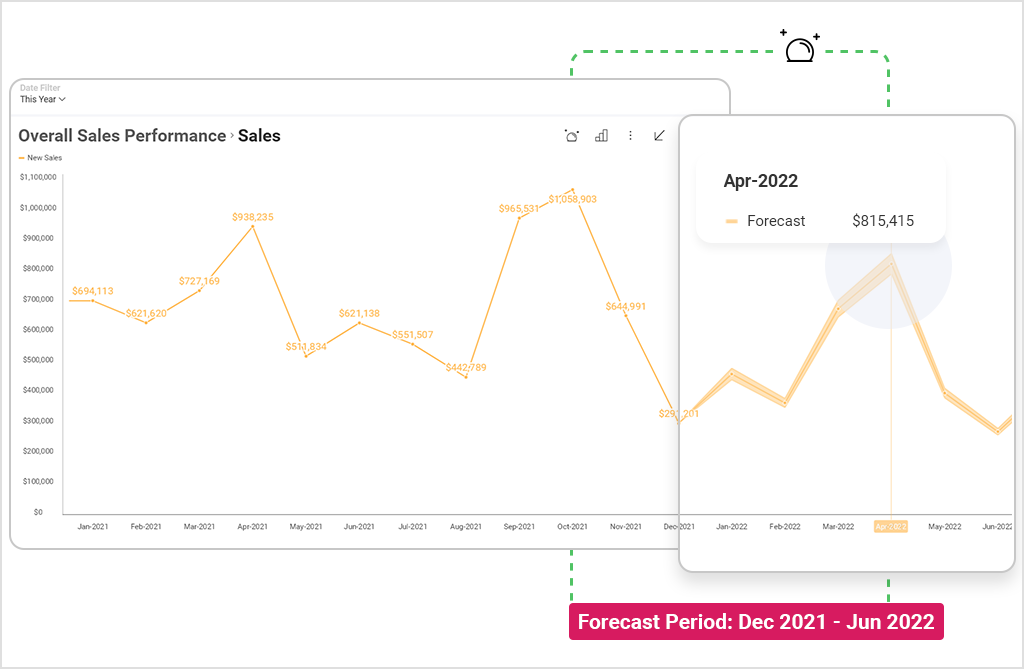
Marketing – marketing teams are using predictive analytics to look at how consumers have reacted to the overall economy when planning a new campaign and use these insights to create and run targeted campaigns that will entice consumers to make a purchase.
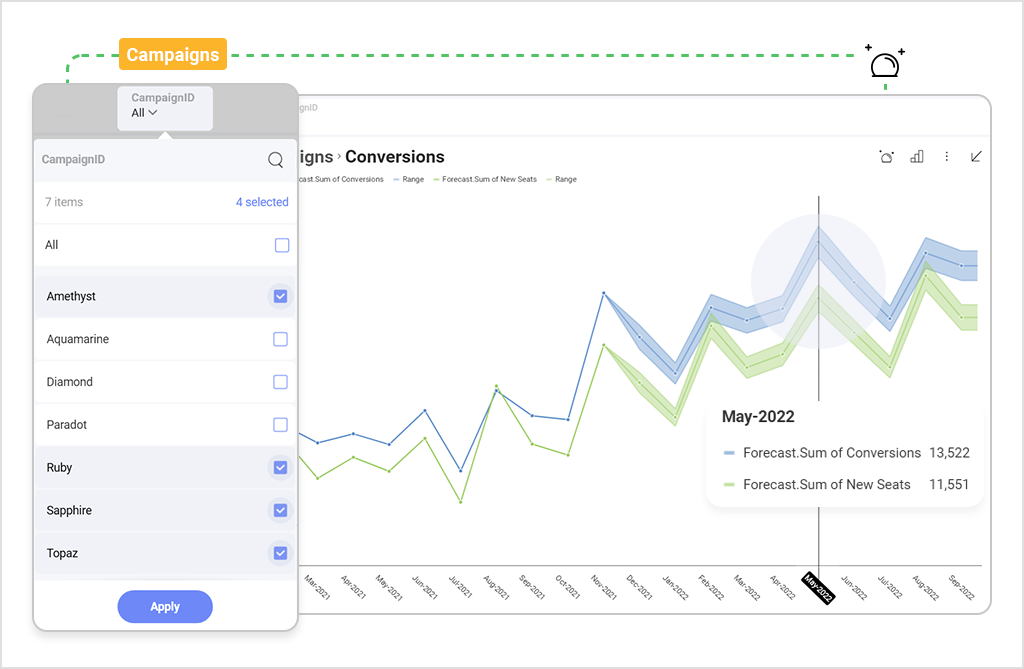
Energy – analytics in power plants can help predict equipment failures and future resource needs, or when power-generating turbines need maintenance. It can also predict when customers might receive a higher bill and send out customers alerts.
Weather – one of the best examples of predictive analytics is weather forecasting. Today’s weather forecasting is possible for months ahead thanks to satellites monitoring the land and atmosphere. They feed that data into models that determine what weather to expect.
Leverage Advanced Predictive Analytics with Slingshot
Data analytics tools simplify the way users process and analyze data, explore the relationships and correlations between data sets, and identify patterns and trends for interpretation. Such a tool is Slingshot.
Slingshot is a tool that aggregates data analytics, project and content management, and chat – all in one, intuitive app. As a tool for data analytics, Slingshot helps users leverage actionable insights at the point of impact. It offers robust advanced analytics functionalities, including machine learning, data blending, dashboard linking, data catalogs, and much more. Thanks to the full business intelligence engine inside it, you can quickly connect to your data and create a beautiful yet informative dashboard with just a few clicks.
With Slingshot, you can accelerate time to insights and unlock your business data’s full potential. Not convinced yet? Try Slingshot for free and see for yourself how it can help your team utilize data easier than ever before.